Introduction
In an era defined by rapid technological advancement, construction - a sector historically seen as resistant to change - is undergoing a data-driven revolution. Projects are being evolved by professionals who rely less upon intuition and experience alone. Today, data is not only shaping the industry but also driving more informed decisions, increasing efficiency and delivering greater value to clients. This shift to data-driven construction is not only streamlining processes but also making projects quicker, more cost-effective and less prone to mistakes and rework.
With rising prices across the sector, the impact of data is becoming even more significant, helping to mitigate inflationary pressures and reduce overall project risk. This transformation is reshaping the industry, making it more resilient and agile in the face of economic challenges.
This article explores how data is informing decision-making, transforming the construction landscape and empowering clients with unparalleled transparency and control.
Setting the Scene: The Current State of Play
In today’s construction industry, data is abundant but often underutilised. Despite the proliferation of information across projects, much of it remains siloed within organisations and disparate systems - such as spreadsheets, site reports, and manual logs - that rarely communicate effectively with each other. This fragmented landscape means that valuable insights often get buried under layers of inconsistent data, requiring significant time and effort to reconcile. As a result, decision-makers often spend more time sifting through information than extracting actionable insights.
While some construction firms are making positive strides in improving their data management, manual data processing is still commonplace, amplifying inefficiencies. Site managers and planners often input data manually, a practice that not only increases the risk of human error but also detracts from more strategic, high-value tasks. For instance, a project manager might spend hours consolidating daily reports instead of focusing on critical issues like supply chain delays or resource allocation. This reliance on manual processes hampers the industry’s ability to respond swiftly to emerging challenges. The construction industry is particularly prone to these inefficiencies due to its fragmented nature, with a multitude of stakeholders working with different systems, each generating data in varied formats. The lack of standardisation and integration between these systems makes automating data collection and management especially challenging.
Moreover, the data that informs decisions is frequently outdated. By the time information is collected, processed and reported, it often reflects yesterday’s reality rather than today’s challenges. This time lag forces construction teams into a reactive stance, addressing issues like labour shortages or material delays only after they’ve become critical. Without real-time data, opportunities for proactive problem-solving are missed and inefficiencies creep into the project lifecycle.
The industry’s limited analytical capabilities further highlight these shortcomings. Most cost management firms rely on basic reporting tools that offer a backward-looking view of performance rather than forward-looking insights. While firms like G&T incorporate market-tested rates into their cost estimates, many rely heavily on historic data, which may not reflect current market conditions. This reliance increases the risk of inaccurate bids and potential overruns. Similarly, safety monitoring systems might generate incident reports days after an event, delaying preventive measures that could avert future accidents.
These challenges illustrate the urgent need for a more integrated, real-time approach to data use in construction - an area where platforms like Asite have already made strides in project-specific data collection and analytics. However, to fully capitalise on the potential of data, the industry must further integrate artificial intelligence (AI) and advanced predictive technologies. By moving beyond current capabilities, such as retrospective reporting, towards truly forward-looking insights, we can transform raw data into actionable intelligence that drives smarter decision-making and empowers clients.
The Shifting AI and Data Landscape
The swift advancement of data-driven technologies, especially those leveraging AI, is transforming industries globally - and construction is no exception.
As projects grow in scale and complexity, so do the demands placed on stakeholders. Modern construction projects involve a vast network of players - clients, contractors, architects, engineers and suppliers - each contributing to intricate timelines, budgets and deliverables. The increasing complexity, coupled with tighter regulatory environments and more ambitious sustainability goals, has created a storm of challenges that traditional project management tools struggle to address. These new demands require heightened transparency, continuous performance data tracking and detailed reporting for regulatory compliance and sustainability targets. As such, the need for accurate, real-time data and the ability to efficiently manage vast amounts of information has never been greater. Here, the value of AI and advanced data analytics becomes unmistakably clear.
AI’s potential lies in its ability to harness vast amounts of high-quality project data to streamline processes, enhance decision-making and mitigate risks before they arise. These technologies can offer insights far beyond what human analysis alone can provide - whether it be optimising schedules, predicting material shortages or reducing costly design errors. The demands of today's stakeholders are shifting towards real-time transparency, faster project delivery and cost certainty—all areas where data-driven AI tools excel.
Yet, the pace of technological development can feel overwhelming. With new tools and platforms emerging constantly, identifying which innovations will deliver tangible results is a significant challenge. It’s been said that AI is not a fixed point - its definitions and capabilities are continuously evolving, often leaving construction firms uncertain about where to focus their efforts and capital. In such an environment, the risk of investing in the wrong technologies becomes a real concern. Many companies are understandably cautions, wary of pouring resources into solutions that may not address their specific pain points or improve their bottom line.
However, for organisations that can cut through the noise and identify the right data tools to leverage their data, the rewards could be significant.
The Role of Data in Construction
As construction projects become more complex and stakeholder expectations rise, data-driven tools are becoming essential for making informed decisions throughout the project lifecycle. Below, we explore how these tools are transforming key stages of the construction process.
Harnessing AI: A Game Changer for the UK's Strained Planning System
One of the earliest and most critical stages of any construction project is design and planning. In the Labour Government’s manifesto, reforming the planning system was a top priority. A recent report[1] from the London Property Alliance (LPA) highlighted that the UK planning system is ripe for a transformative leap, driven by smarter use of data. With over 3.5 million planning applications processed each year by 337 local authorities[2], the system is under severe strain. The manual handling of these applications is not just laborious, it is a bottleneck that stifles new development.
Our planning system has yet to fully tap into the potential of digital tools and data management practices, let alone the transformative possibilities of AI. While reluctance to embrace technological advancements plays a role, other factors such as constrained public funding, lack of capital investment, human resource, and inconsistent leadership are equally significant. These challenges prevent the system from modernising and streamlining the drawn-out planning process. The lengthy approval timelines that result are prohibitive for many developers, especially those holding land in high-cost urban areas while awaiting decisions.
But the UK planning system doesn’t have to be like this. AI stands poised to revolutionise the planning landscape, offering the potential to automate laborious tasks, accelerate the application approval processes and refine decision-making. Currently, planning documents must be submitted in PDF format, forcing planners to sift through them manually to extract key information. However, there are calls to start treating planning applications as data rather than documents. By moving away from unwieldly PDFs and processing applications in more data-friendly formats, planning authorities could dramatically cut down the time spent interrogating documents. Imagine local councils harnessing AI to tackle technically complex proposals - such as whole-life carbon assessments - even with limited in-house expertise at their disposal.
Yet, for AI to reach its full potential in planning, the system must change not only how it handles applications, but also the way it stores planning data. Datasets will need to be centralised and open – not dispersed and siloed, as they currently are. Without these changes, we risk entrenching a two-tier system, where only well-resourced local authorities can reap the benefits of AI integration. Access to resources, shared data curation and strong government backing will be essential to ensure that the transformative power of AI is shared equitably across the planning sector.
There have been some positive developments in this space. The City of London is in the early stages of developing an AI-driven 3D visualisation tool that can explain complex planning policies in clear, jargon-free language. This tool aims to make regulations more accessible to a broader audience, including the public and stakeholders. Additionally, there is potential for AI tools to scrape relevant data from planning portals and local authority websites. AI "bots" could be programmed to query the planning data, answering specific questions about planning applications and policies. This would allow users to access vital information quickly and efficiently, without the need for deep technical expertise, streamlining the entire planning process.
Enhancing BIM: Transforming Static Models into Intelligent Systems
Building Information Modelling (BIM) has long been a cornerstone of data-driven construction, influencing decisions from the earliest planning stages through to project completion. In the initial phases, BIM facilitates site selection, feasibility studies and regulatory compliance by aggregating diverse data sources into a cohesive model. As projects progress into the design phase, BIM integrates various data streams into detailed 3D models, enabling stakeholders to visualise and analyse every aspect before construction begins. This approach not only enhances design accuracy but also optimises processes by simulating scenarios and predicting outcomes. By identifying and resolving potential issues early, BIM reduces both time and costs across the project lifecycle.
While we’ve explored the potential of AI in BIM in previous articles, recent advancements in AI are significantly amplifying BIM’s capabilities, transforming it from a static modelling tool to a dynamic, predictive platform. AI-driven generative design, for example, allows BIM software to create optimised design solutions by analysing vast historical datasets. AI-enhanced clash detection can proactively identify and prevent design conflicts before they become costly on-site issues. Additionally, AI is improving predictive maintenance through real-time data integration from IoT devices, allowing for continuous monitoring and more effective building management post-construction.
Moreover, AI tools within BIM are increasingly being used to optimise designs for sustainability, integrating carbon footprint analysis. AI algorithms can analyse large datasets to calculate the environmental impact of material choices or design modifications, enabling real-time tracking of a project's carbon footprint and identifying ways to reduce emissions throughout the building’s lifecycle.
On the safety front, AI-driven analysis is leveraging historical data to predict potential risks, creating safer construction environments. Natural language processing (NLP) has also made its way into BIM platforms, enabling stakeholders to query complex project data more intuitively, asking questions in plain language (eg “What’s the expected completion date if we change this material?”) and AI interprets these queries to extract relevant data and provide responses. This functionality broadens access to critical project information, streamlining communication and decision-making across teams.
While AI integration into BIM offers substantial benefits, including predictive capabilities and automation, it also introduces risks related to data quality, standardisation, operational complexity and security. Addressing these risks requires careful oversight, high-quality data management and ongoing human involvement.
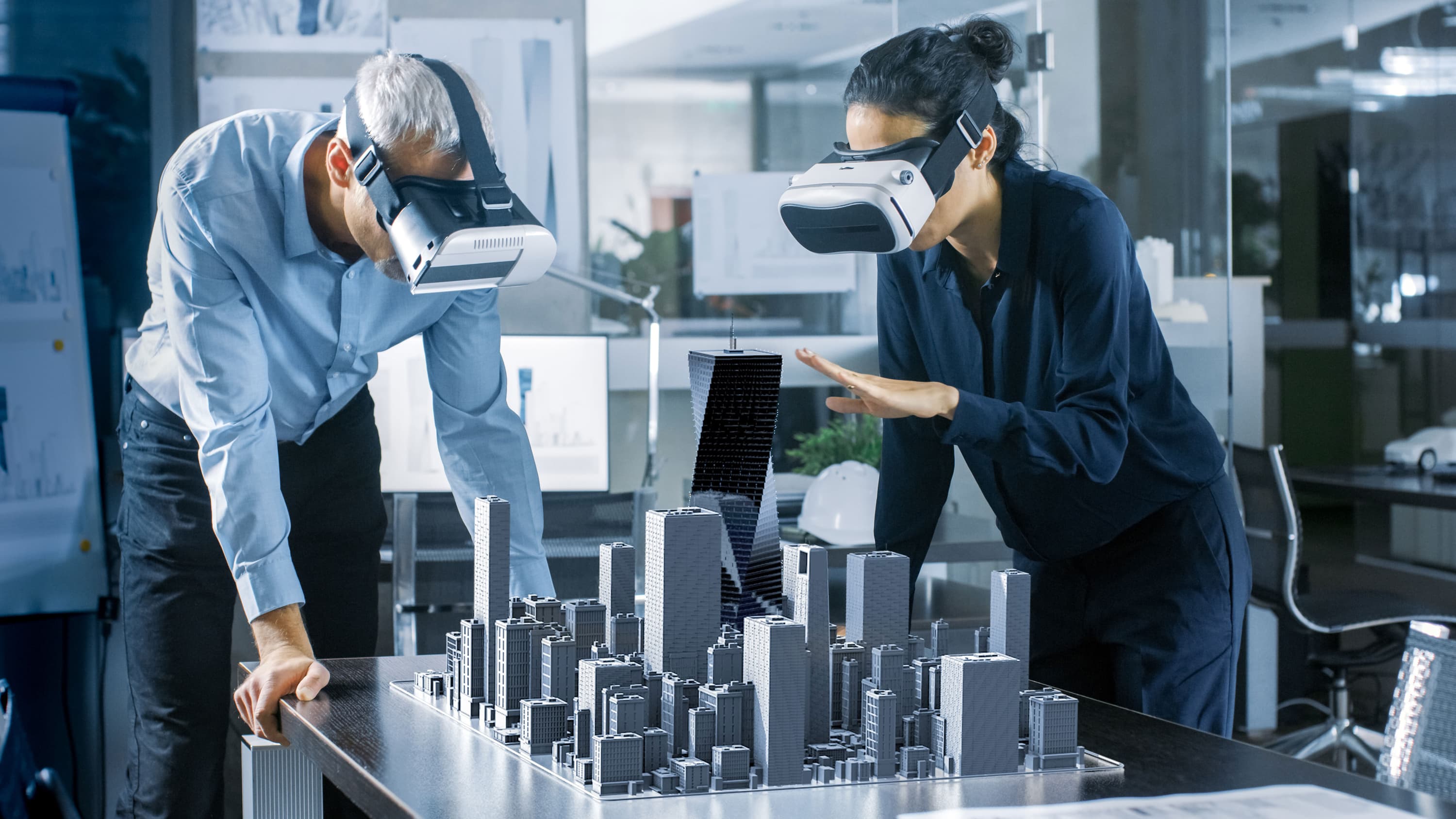
Predictive Analytics: Revolutionising Risk Management and Project Precision
Beyond BIM, predictive analytics tools, such as those offered by nPlan, are transforming the way we forecast project deliverability and manage risk. By leveraging historical data from past projects, these algorithms can predict schedule delays with remarkable accuracy. With hundreds of variables influencing project timelines - from activity descriptions to less obvious factors, such as reduced productivity levels on Mondays[3] - AI can uncover patterns that human intuition might overlook. This allows project teams to focus their attention on the most critical areas of a project, reducing the likelihood of unforeseen disruptions.
However, data alone isn't a silver bullet. The real challenge lies in filtering out bias and noise (ie irrelevant information) to ensure the data driving decisions is truly meaningful. The human brain isn’t equipped to process the immense volume of data generated by complex projects, often making even the most basic algorithm a more reliable guide than gut instinct. Our cognitive limitations can lead to misjudgements, such as proximity bias - where we overestimate risks we’ve recently encountered while underestimating less obvious but equally critical risks. This is where AI shines - by working beyond human biases, it sifts through vast data sets to reveal trends that may not be immediately apparent to human decision-makers.
It’s a popular misperception that "more data is always better”. In reality, the truth lies in balancing data quantity with quality. However, even in large datasets with some flawed inputs, algorithms can still identify reliable trends, provided poor-quality data remains a minority. AI tools are designed to optimise based on the majority of relevant data, using performance-led indicators to weed out less useful information. This is critical in industries like construction, where vast amounts of data are generated but are often fragmented, inconsistent, incomplete or outdated.
Cost, which can be notoriously difficult to predict accurately, is another crucial variable in project outcomes. AI can help bridge this gap by offering a level of predictive power that surpasses traditional models. However, unlike analysing data in Excel, which allows for full transparency and traceability of every calculation, AI-driven models can be far more complex and often operate as “black boxes”. This opacity can make it difficult to explain or validate how certain predictions are made. It can also make decision-makers, who are accustomed to having full visibility and control over the analysis process, uncomfortable.
It is crucial to recognise that these AI tools are designed to augment, not replace, human expertise. While algorithms excel at processing vast amounts of data, they lack the nuanced understanding that only hands-on experience provides. Human professionals play a crucial role in questioning assumptions, scrutinising AI-generated outputs and applying their expertise to project-specific interactions that algorithms might miss – the so called “bookending” approach[4]. AI is effective in presenting a range of potential outcomes, but it is ultimately human decision-makers who must interpret these insights and apply them to practical, real-world scenarios.
We should therefore treat current AI tools as valuable decision-making compasses, not panaceas. They help us navigate the complexity of modern construction projects, offering insights that can lead to better outcomes. However, we’ll still need the expertise of people to ensure that those insights are applied effectively.
Procurement and Supply Chain
Procurement decisions and supply chain management are also becoming increasingly data-driven, with AI playing a critical role in shaping efficiency, cost control, and risk mitigation. The integration of vast datasets and advanced analytics is transforming traditional approaches, offering both opportunities and new challenges for the construction industry.
Data-Driven Procurement: Enhancing Efficiency and Mitigating Risk
Insolvencies are an ever-present risk in construction. As we’ve seen, even well-established main contractors can fail with little external warning, leaving stakeholders and project schedules hanging in the balance. However, AI-powered systems may be able to help mitigate this risk by optimising the supplier selection process and identifying any early signs of financial instability.
Traditionally, supplier evaluation relied on manual data collection and analysis, which was both narrow in scope and prone to human bias. In contrast, AI algorithms can analyse far more data points, ranging from performance metrics and delivery history to customer feedback and compliance with contractual terms. This capability allows for a more comprehensive and objective assessment of supplier reliability and suitability, with algorithms identifying trends and patterns in the data that may not immediately obvious to human evaluators.
For instance, Dun & Bradstreet’s AI-driven platform evaluates the financial stability of contractors by tapping into a wealth of B2B, public, proprietary and business activity data. Its advanced models create financial health ratings and risk scores, helping companies predict the likelihood of contractor insolvency. This predictive power is especially critical in high-risk sectors such as construction, where delays or contractor failures can lead to significant project disruption. Recently, Dun & Bradstreet partnered with IBM to create D&B Ask Procurement, an AI assistant that offers predictive analytics on supplier reliability. The tool can integrate directly into enterprises’ existing procurement systems which house their own data and insights on suppliers. By automating many of the traditionally manual tasks involved in supplier evaluation, D&B Ask Procurement aims to streamline access to procurement data, optimising the processes and reducing associated risks.
While G&T does not advise on the financial stability of contractors or the supply chain, it has utilised Dun & Bradstreet’s solutions to assist some clients with contractor selection and procurement strategy. This use of data-driven insights supports more robust decision-making, mitigating risks associated with procurement and fostering smoother project delivery.
Beyond evaluating contractors and their potential risk of failure, other tools have been developed to assist with various aspects of procurement, including demand forecasting, bid evaluation, contract analysis and management as well as materials selection and availability.
Predictive Analytics: Revolutionising Budgeting, Forecasting, and Tendering
The integration of AI into budgeting, forecasting and tendering processes has the potential to enhance how construction firms handle cost estimation and project planning. By analysing historical data alongside real-time market trends, predictive AI helps firms to anticipate fluctuations in material costs, labour demands and overall project expenses with greater precision. This results in more competitive tenders that better reflect current economic conditions and market dynamics, minimising the costly risks associated with under- or over-bidding.
For example, Autodesk Construction Cloud (ACC) employs AI-driven analytics to provide real-time assessments of material costs, factoring in variables such as supply chain disruptions, regional market fluctuations, and sustainability considerations. This gives contractors a tactical edge, enabling them to submit more competitive bids without compromising their profit margins—an essential capability in today’s inflationary environment. ACC’s AI models also analyse historical project data to identify trends in material and labour costs, projecting these forward to enhance the accuracy of cost estimates during the tendering process.
Similarly, tools like Procore and Trimble’s WinEst incorporate predictive models layered on top of historical datasets. These tools assess the probability of cost overruns or project delays based on patterns observed in past projects, allowing construction managers to build risk contingencies into their budgets. This level of foresight dramatically improves cost accuracy during the tender phase and reduces the likelihood of unexpected, expensive issues arising later in a project.
AI’s ability to analyse competitive bid data also is also reshaping pricing strategies in tendering. Platforms like ConWize leverage AI to optimise the tendering process by analysing historical bid data, market trends and project specifics. The platform automates bid preparation, reducing human errors and helps contractors identify the most competitive pricing strategies based on current market conditions. For firms with limited bidding capacity, this technology can quickly generate accurate bids with minimal resource strain. This enables firms to bid more efficiently, freeing up critical resources for other strategic tasks. As these tools continue to evolve, they won’t just provide a competitive edge - they will reshape the entire tendering landscape, with success increasingly dependent on the strategic insights AI can uncover from vast data troves.
AI’s entry into construction is not just about keeping budgets tight - it’s about fundamentally reshaping how businesses approach risk, competitiveness and long-term planning. Predictive tools will give forward-thinking firms the ability to plan in ways that traditional methods simply can't. As the technology continues to mature, its impact will extend far beyond individual tenders, altering the entire realm of construction finance and strategy.
Enhancing On-Site Performance with Data-Driven Technologies
Data has also become a critical asset in the dynamic environment of on-site construction, driving improvements in safety, efficiency and decision-making. Real-time data collection through Internet of Things (IoT) devices - such as sensors, drones, and cameras - enables contractors to monitor site performance continuously. Wearable sensors, for instance, can track workers' movements to ensure safety compliance, while drones provide aerial views to assess progress, identify hazards and measure earthworks with exceptional precision.
This flood of real-time data feeds into Digital Twin technologies, creating a dynamic, digital replicas of physical construction sites. These virtual models, continuously updated with live data, allow managers to make real-time adjustments to resource allocation, equipment deployment, and even on-site traffic flow. Industry leaders like Skanska and Royal BAM Group have embraced Digital Twins to optimise processes ranging from equipment usage to material delivery scheduling, reducing delays and enhancing resource efficiency.
Integrated platforms such as Procore, PlanGrid, and BIM 360 capture and consolidate data directly from construction sites. These tools offer a holistic view of cost control, supply chain management, and sustainability metrics. By linking these live data points to cost projections, project managers can quickly identify any discrepancies between planned and actual expenditure, enabling more informed and proactive decision-making. Similarly, construction firms are now using these insights to track their environmental impact, monitoring energy usage, emissions and waste in real time to meet increasingly stringent sustainability targets.
Emerging AI applications are pushing the boundaries of site management. Buildots, for example, employs computer vision and AI through 360-degree cameras mounted on hardhats to automatically capture site progress during routine walkthroughs. AI algorithms then analyse the footage to generate detailed progress reports, comparing actual conditions against BIM models and schedules. This automated system flags any deviations from the plan, allowing project teams to address issues early and adjust timelines accordingly – reducing the risk of costly delays.
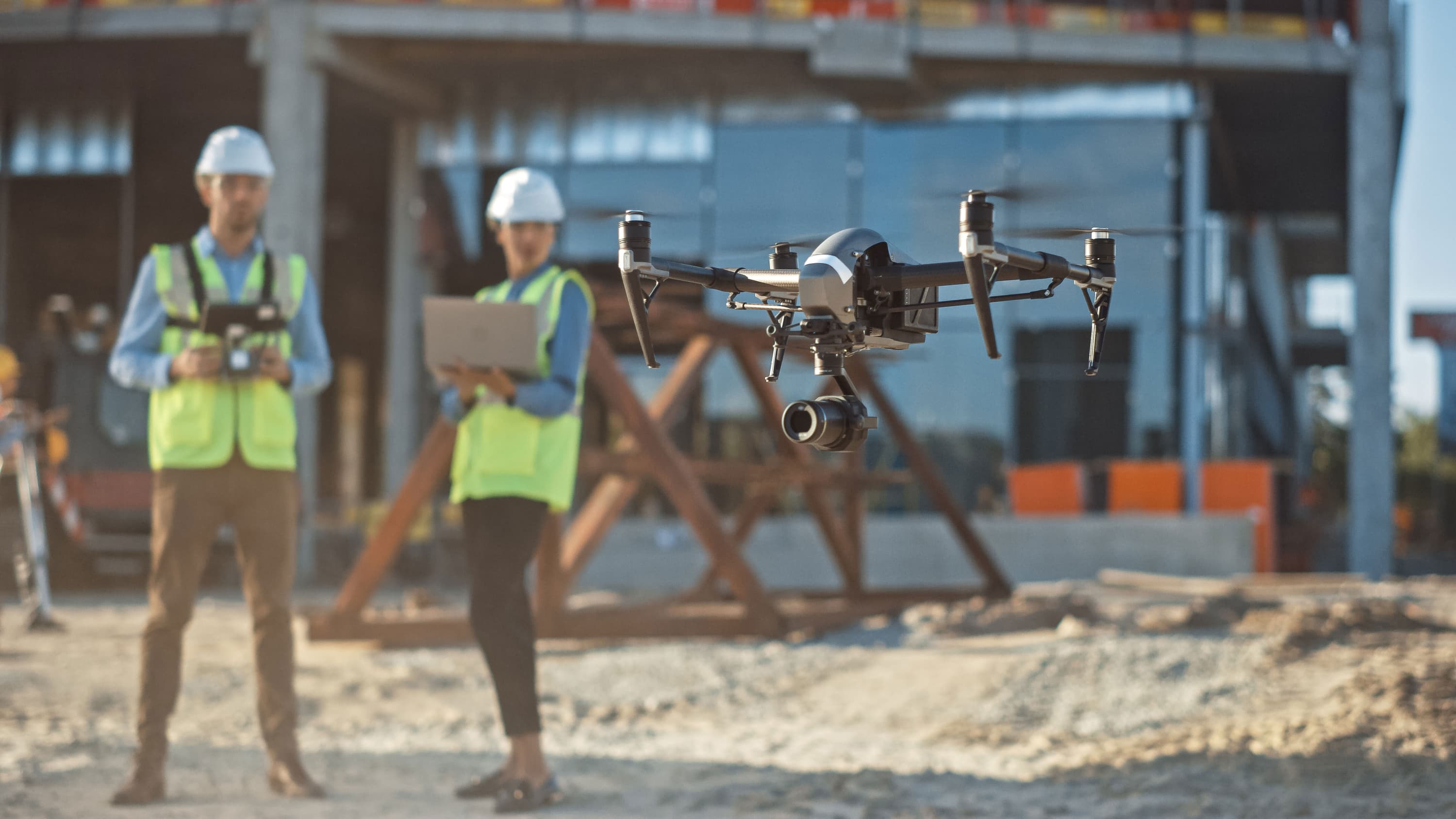
The Vital Role of Human Expertise in AI Integration
While these technologies represent the future of construction management, human expertise remains indispensable. AI certainly has the transformative potential to shift expertise from repetitive, resource-intensive tasks to creative problem-solving. After all, no one went to university to compile snagging lists. The hope is that AI can and will handle these less desirable tasks that drain on the intellectual capital of highly trained experts who have invested years in developing sophisticated skills.
At its core, AI excels at processing vast datasets and identifying complex patterns. However, it currently lacks the ability to make more nuanced judgements based on contextual understanding, emotional intelligence and holistic reasoning. High-quality data and well-defined parameters, provided by humans, are essential for AI’s effectiveness. We must train, guide and rigorously verify these systems to ensure reliable outcomes. Moreover, AI is not yet fully capable of understanding broader socio-economic contexts, where statistical analysis falls short of capturing the dynamic subtleties of human systems. Here, human judgment remains indispensable.
These data-led technologies should therefore be viewed as another lever to pull – a new tool to embrace. Their full potential depends on a balanced partnership with human expertise. The challenge is for us to work out how to best add value beyond the capabilities of the technology itself.
G&T’s Smart Data Platform: Leveraging Project Insights
In our journey to use data more efficiently, G&T has developed its own tools and platforms to leverage the vast amount of project data we hold. After standardising our data, ensuring its structure and format is consistent across the business and collecting it in a central database, we created G&T’s bespoke Smart Data platform. The platform enables us to carry out a range of analyses, track emerging trends and generate insightful benchmark reports.
G&T’s visually rich benchmarking tool showcases the various components of the build, allowing us to produce bespoke, interactive and informative benchmarking reports for our clients. These reports can be high level, broken down into different project elements. They can also benchmark projects against similar ones based on various characteristics. Feeding into these reports is G&T’s market leading TPI analysis, which allows us to compare past projects with real-time cost information.
While the tool’s primary purpose is to streamline data collection and comparison – simplifying the historically laborious process of creating benchmark reports – organising our data in this way will allow us to capitalise on emerging data and AI technologies more effectively.



G&T’s Smart Data tool is one of many data-driven solutions we have deployed. As a business, we are investing in a range of tools and solutions to leverage the vast amount of project data we possess. Our market intelligence tools are becoming ever more sophisticated, enhancing our core publications – such as our TPI and Market Update reports – as well as our client services.
To find out more about G&T’s growing data capabilities and market intelligence tools, please get in touch.
References
[1] https://www.londonpropertyalliance.com/ai-and-the-built-environment/
[2] Ibid
[3] https://www.forbes.com/sites/tracybrower/2023/08/13/productivity-plummets-on-mondays-how-to-be-your-best-all-week-long/
[4] where the human initiates and concludes the process