In its 2020 report, The Next Normal in Construction: How Disruption is Reshaping the World's Largest Ecosystem[1], McKinsey identified a growing focus on solutions that incorporate artificial intelligence (AI) to enhance both the quality and efficiency of construction projects. AI in construction has the potential to add value across the entire project lifecycle and construction ecosystem, from the early stages of project design, tendering, financing, to procurement and construction. It even has a role to play in the operational and asset management side.
Some of the biggest and most enduring challenges currently faced by the industry, including labour and skills shortages, cost and schedule overrun (due to a number of possible reasons such as a change in requirements) and productivity and safety, can be addressed by AI - and with barriers to entry steadily falling, the technology is set to play an increasingly important role.
What is the goal?
AI can take over monotonous, cumbersome and repetitive tasks, help with design and planning and allow construction teams to dedicate more of their time to honing their expertise and creativity.
Some of the key high-level goals of AI and Machine Learning (ML) in construction include:
1. Reduce human error and costly rework
- Data entry workflows can be a time-consuming process and, like all manual tasks, is exposed to the potential risk of human error. AI/ ML can automate many of these labour-intensive tasks (eg log creation and document closeout)
- Utilising advanced automated processes can flag up errors before they continue downstream and result in costly rework
2. Mitigate risk
- Financial risk – inaccuracies and miscalculations can delay projects and result in rework costs, but AI/ ML can put certain tasks on autopilot, reducing uncertainty and mitigating design risks
- Physical risk – risks can be flagged before works starts on site because technologies can understand critical quality problems, provide visibility into high-risk areas and even take action to help mitigate those safety behaviours and hazards
3. Save Time and Free Up Focus
- While human input and expertise will still be necessary, AI/ML tools can enhance quality control and speed of work through greater traceability and fast deep learning systems, freeing up time for the more important, strategic elements of the construction process
Humans will need to work in tandem with new AI technologies which will create new opportunities and occupations to operate and oversee the work of AI-based solutions. Due to the inherent complexity of construction work, construction has always been and will largely remain a human business. Unlike manufacturing, which is characterised by repetitive tasks that are relatively easy to automate, many tasks within the construction sector, both on and off site, currently remain beyond the capabilities of AI, machines and robots. Each trade involves a myriad of intricate tasks, and no single project is exactly the same as the last one in construction, which makes automation far more difficult on a relative basis.
AI technologies will need human intelligence in order to be most effective and the best productivity gains will only materialise as people work alongside machines. McKinsey suggests that any workers that are displaced by automation following the transformation of business processes, will find other employment through the creation of new types of construction jobs. [2] Yes, new skill sets will need to be learnt and additional training will be required for these positions, but we have experienced this in the past with other technological advancements across many sectors.
While many tasks within the construction sector, both on and off site, remain beyond the capabilities of AI, below we explore some of the broad potential benefits to the sector and consider how AI-based solutions might help cost and project managers become even more effective.
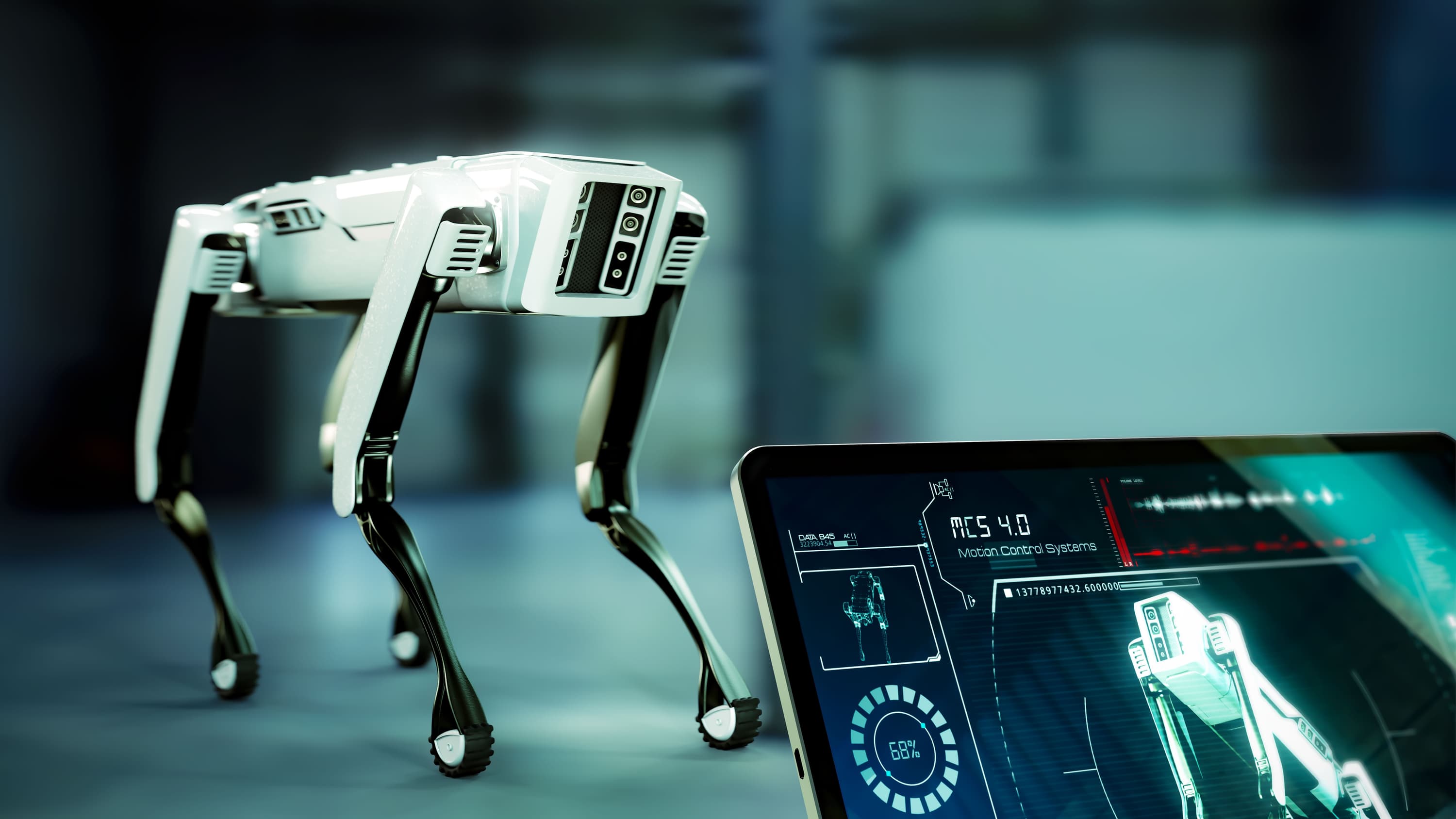
What can AI do in construction?
The potential applications of machine learning and AI in construction are vast. There are several applications already is use, including:
- Prevent cost overruns: the use of ‘Artificial Neural Networks’ can predict cost overruns based on factors including project size, contract type and experience level of project managers. Historical data is used to create predictive models to generate realistic timelines and costs for future projects
- Better, generative designs: AI-powered generative design can identify and mitigate clashes between the different 3D BIM models generated by different teams. Software uses ML algorithms to explore all variations of a solution and suggests alternative designs based on constraints
- Monitor risk: contractors are already using ML solutions that monitor and prioritise risk on site. The software can automatically assign a priority level to any issues and rank subcontractors based on a risk score to help mitigate risk
- Project planning path: robots can autonomously capture 3D scans on site and the data is fed into a deep neural network which classifies how much progress has been made on sub-tasks. Management is notified if the project is off track so the problem can be dealt with before it escalates into a major issue. Algorithms learn from trial and error by assessing endless combinations and alternatives based on similar projects, correcting itself over time
- Site productivity: self-driving machinery can efficiently perform repetitive tasks such as concrete pouring, demolition and bricklaying. Semi-autonomous bulldozers can prepare sites to exact specifications, freeing up human workers for other construction work and reducing project completion times
- Scanning for safety hazards: some companies have created algorithms that analyse photos from construction sites, scans them for safety hazards and correlates the image with historical accident records. Risk ratings are computed when an elevated threat is detected
- Labour shortages: AI can address labour shortages and low labour productivity levels by evaluating job progress, the location of workers/equipment so project managers can determine whether a site has enough workers/equipment to complete the project on schedule. Real-time analysis detects when a project might be falling behind schedule and when additional labour could be deployed
- Off-site manufacture: Staffed by autonomous robots, off-site factories can assemble components of a building (eg assembly-line walls) more efficiently than humans can on site, leaving more time to focus on detailed work (eg HVAC and electrical systems)
- Post-construction: Information collected through sensors, drones and wireless tech is used by analytics and AI-powered algorithms to produce operational and performance-based insights about the building. Developing problems are monitored and preventative maintenance schedules can be established
AI can scrutinise mountains of data, including requests for information, open issues, change orders, equipment and worker monitoring, materials delivery, claims management, progress tracking and production analysis. Critical things can be flagged up, highlighting the levers to pull so as to improve outcomes.
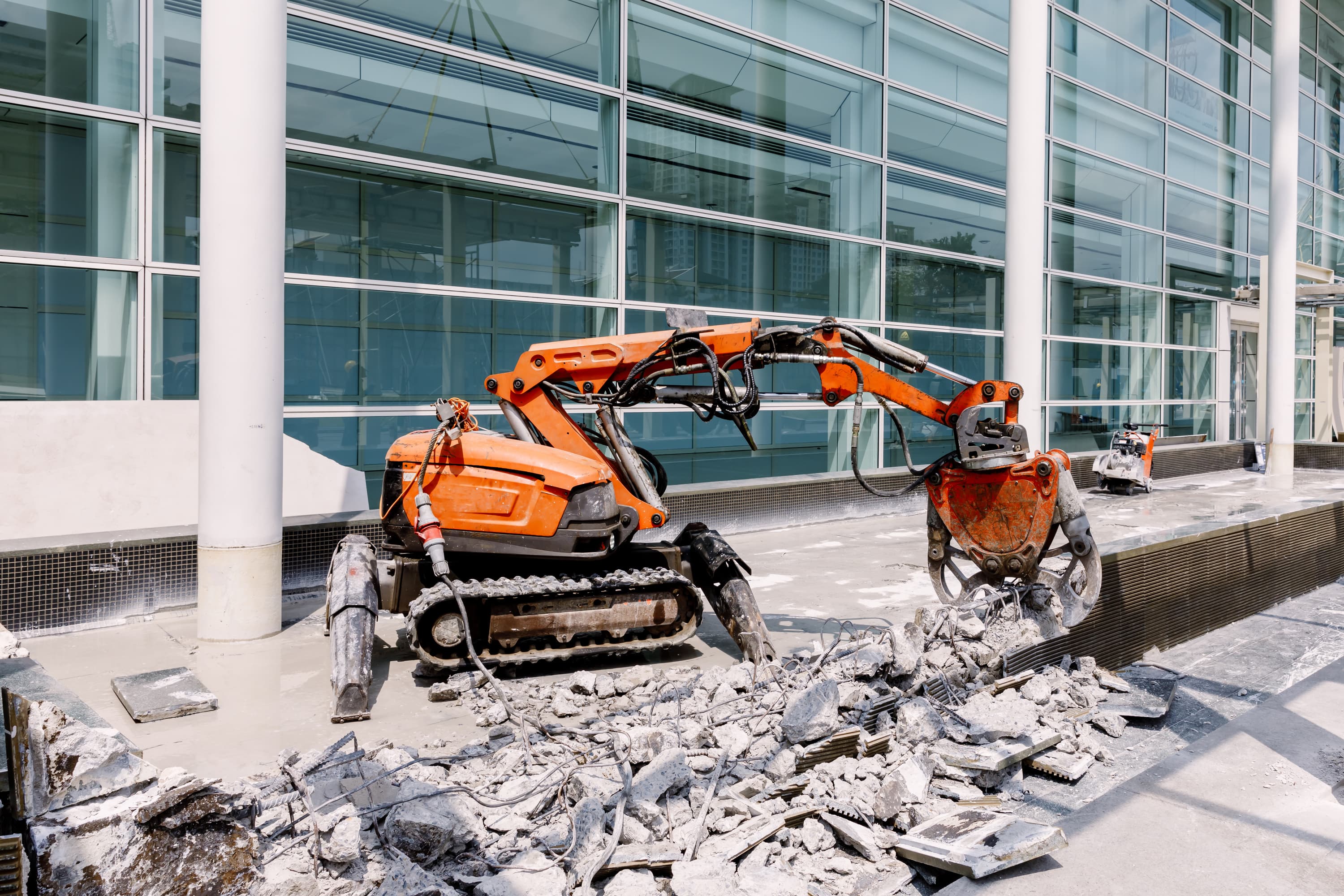
Application to Quantity Surveying
When cost estimating in the absence of quality information during the early pre-tender stages of a project, historic data, past experience and knowledge is relied on when preparing the initial cost estimate, but there are a large number of interrelated variables in the construction process and computerised cost estimating algorithms have the potential to improve estimating accuracy at the preliminary stage of projects.
BIM (or Building Information Modelling) has already enhanced the built environment landscape with its ability to automate certain measurement tasks, increasing both speed and accuracy. BIM has taken the industry a long way since it was first introduced in the early 2000s, but could AI improve BIM systems further? A number of BIM software companies have already begun to use AI to improve efficiency, but we are likely to see much more AI-assisted BIM over the next decade.
BIM software can now use ML to study, mine and detect patterns in cost data and, from this, make independent decisions on how to automate and improve the model building process. Once a database of ’useful’ cost data has been established – something which should be quite plausible due to the huge amounts of data the industry generates and is collected by BIM software – ML techniques can be employed for clustering, prediction, and classification tasks. From here, the collected and organised data can be used to extract possible causes of cost or time overruns, which will ultimately provide clients more value for money.
Future BIM progressions could potentially carry out a quantity take off[3] of materials with accurate descriptions in a Bill of Quantities, or automatically highlight problems on jobs before they arise using historical data. AI-assisted BIM systems would help to see project progress in near real-time so when one aspect of the building is updated and fed into the system, all other aspects are automatically updated without the need to inform of change everything individually.
Simply put, BIM software has the ability to collect and work with a range of construction data, but it is AI that will help make sense of this data. Through analysis, predictive and model-making capabilities, more efficient solutions can be unearthed.
To summarise, AI augments surveyor productivity, helps keep a consistent project and version story, communicates structural changes in requirements to clients and helps coordinate work between teams. However, while using these AI tools will help the surveyor get to a percentage of the story, expertise and judgement will be necessary to correct any incorrect assumptions made by the AI. Those that embrace these changes will be better able to provide their clients with high-value, strategic services. According to Nick Rowe, Partner & Board Member at Gardiner & Theobald:
“AI can help tackle many of the current challenges in the construction industry, allowing the sector to evolve and grow at a faster rate. AI technologies will certainly be part of the QS’s toolkit in the future. Coupled with the expertise, judgement and wide-ranging skillset of a human QS, we will be able to create a powerful combination of insights to benefit our clients’ projects.”

Application to Project Management
AI is already being used for administrative project management tasks, but it is now evolving to handle more complex tasks. A few examples of AI-focused approaches and solutions on the horizon include:
- Project schedule optimisation: AI-based algorithms can process project parameters and consider numerous alternatives for project delivery schedules, suggesting possible sequences, durations and any associated work costs to ensure project delivery is on-time and on-budget
- Transport route optimisation: Available AI technology can already optimise routes and improve traffic navigation, but emerging techniques could further optimise by assessing endless combinations and alternatives, solving for objective functions such as duration and cost of fuel. This allows PMs who want the least cost routing to work out the logistics of having material supplied on-site
- Supply chain optimisation: supervised learning applications can enhance supply chain coordination. AI can help navigate longer and increasingly interlinked physical flows of materials by enabling end-to-end transparency, creating demand forecasting models and automating the physical flow of materials
- Image recognition/classification: images and video taken on site can identify safety issues to inform future approach and training to help avoid unsafe behaviours
- Real-time analytics: AI platforms can collect and analyse data from sensors to alert project managers about critical items that need their attention. It can also help PMs to understand patterns in relation to maintenance and unplanned downtime as well as deploy real-time solutions
- Constructability and risk detection: Predictive AI solutions can forecast project risk, constructability, as well as the structural stability of various technical solutions. Having these insights during the key decision-making phases of a construction project can reduce costs
- Quality control: deep-learning techniques can analyse captured images and compare any construction defects against existing drawings
The number of potential AI solutions applicable to construction project management are endless.
Labour resource planning is another area that ML can tackle, with technology helping to segment employees based on likelihood of attrition. The algorithms can also identify potential candidate pools, locate and predict talent pain points such as availability and skill level, find flaws in organisational design and help tailor recruitment strategies to attract the right talent. All of this can help with project planning and limit costs and project delays.
ML applications also extend to sentiment analysis and profiling of suppliers. After several globally disruptive events in recent years, confidence in suppliers’ ability to meet their commitments has dropped and clients are naturally concerned. ML algorithms can conduct sentiment analysis on suppliers, assessing their reputation for meeting commitments, reassuring clients and helping them make tendering decisions.
Despite its wide range of uses in construction project management, AI will not be a ‘project management terminator’. Human-centered skills such as creativity, perceptiveness and people management are fundamental to delivering a successful project – tasks which AI typically struggles with. While AI may, for example, forecast deviations in programme schedule, it cannot provide a solution for them, nor can it resolve the conflicts created by the deviation or garner the stakeholder consensus needed to get the project back on track.
But by taking on routine, data-driven tasks, AI can free up project management teams to focus on core areas such as people management, project vision and team building. Ultimately, AI is a tool to facilitate productivity. Staying updated with the latest AI applications in the project management space and engaging with them will ultimately complement project management professionals – not supplant them. James Angus, Partner & Board Member at Gardiner & Theobald said:
“As AI evolves to take on more routine project management tasks such as data collection, tracking and reporting, the role and focus of the project manager will invariably see a shift. Inherently human skillsets of creativity, ideation and problem solving will be essential to compensate for the areas where AI technologies fall short. Some business-as-usual tasks may become automated, but soft skills such as communication, negotiation and the ability to collaborate with teams will only increase in value. We see AI acting as a virtual partner to project managers, both working together to increase efficiency and effectiveness while reducing cost and effort.”
Our case study below outlines how project teams at Mace are using AI tools to predict schedules more accurately, measure project progress and mitigate risks and delays.
Case Study: Mace Using AI to Capture Digital Records on Projects
Working with a start-up called ‘Disperse’ – a provider of AI-powered building productivity systems – Mace has captured digital records on a number of its projects. 360-degree images are taken regularly, captured by operatives walking around sites. These images, which are used to create a layered digital twin of a building enabling teams to visually walk through every space on the project, are then uploaded to the Cloud for processing using proprietary AI technologies. Disperse’s building productivity management platform has allowed Mace to:
- Measure project progress and automate progress reporting
- Flag up any discrepancies between what the construction management team and Disperse tell Mace
- Offload necessary admin from the project team
- Allow the project team to work with facts
- Create an enhanced/shared understanding of the project between staggered teams, eliminating confusion and improving the construction workflow
One Crown Place, a mixed-use scheme in the City of London, was one of Mace’s earliest projects to use the Disperse system. It allowed Mace to see what was happening in different areas on site, highlighting any differences from the design and giving progress updates against the programme. However, setting up the technology takes time as the algorithms need to be taught what they are looking at. Mace and Disperse had to identify the required information from drawings and BIM models. Furthermore, because Disperse is not a 100% AI-driven technology, humans are involved in the checking process.
Disperse’s technology was particularly beneficial during the pandemic. Mace’s home-based project teams were able to continue to work without needing to be on site. Valuations, quality checks and sign-offs could all be conducted, as well as planning/re-planning with Mace’s trades. Disperse’s software was also used to guide and inform conversations with subcontractors about reopening sites safely during the pandemic. Mace subsequently made changes to processes to help relevant sites return to a high rate of productivity.
The technology allows Mace and its trades to predict schedules more accurately, to forecast and to mitigate risks and delays. It is also useful for record keeping as it allows Mace to go back to the first time the job was scanned to see how the building unfolded.
In connection with this, Mace is starting on work that will enable it to monitor the new ‘golden thread’ requirement of building safety information. Under the Building Safety Act 2022, companies now need to gather end-to-end information on high-risk residential projects and kept as digital records to demonstrate what has changed in the construction process. Mace is now working with companies to address how they consider capturing quality information for their records.
Mace is trying to digitise all aspects of project delivery so it can then start to apply AI and ML over the top of the captured data. According to Matt Gough, Mace’s director of innovation:
“The challenge that we’ve got as an industry and within our own business as well is that we talk about being data-rich and knowledge-poor, but the reality is we’re not even that data-rich. The sort of volume of data that you need to appropriately apply artificial intelligence and machine learning doesn’t really exist in the construction industry, so that’s why we’re working with some start-ups specifically in this space.”
Conclusion
Construction may have been slightly behind the curve in terms of implementing AI-based solutions, but a shift is coming. There is evidently a growing focus on incorporating AI-powered technologies, as stakeholders across the project lifecycle start to allocate more resource to build AI capabilities.
Early-stage adopters of AI have seen significant benefits already and are taking steps to identify additional areas where AI can have the most impact. Because AI algorithms are based on good data, those firms that have the largest volumes of good quality data, or at least a ‘critical mass’ of data to help train its AI algorithms, will see the greatest short and long-term benefits. Naturally, it will likely be the largest, well capitalised construction companies and professional consultancies that will lead the charge and leverage their data to the greatest effect.
We have seen that AI, when correctly combined with the intricate knowledge of skilled cost estimators and project managers, can create a powerful combination of insights to help clients. One of the key benefits of AI is the early detection of project risks so that they can be mitigated. As indicated by the famous MacLeamy Curve, the earlier you identify problems in a project, the lower the impact they have on cost and margin.
Without doubt, AI will disrupt traditional workflows and incite business process change, but this disruption will be necessary to improve the long-term productivity growth of the sector.
The next article in G&T’s AI series will consider how to best prepare for AI-based construction technologies. It will explore the steps that should be taken prior to deployment of AI solutions and some of the questions that need to be asked, addressing issues such as data discontinuity, inconsistency and inaccuracy.
[1]https://www.mckinsey.com/~/media/mckinsey/industries/capital%20projects%20and%20infrastructure/our%20insights/the%20next%20normal%20in%20construction/executive-summary_the-next-normal-in-construction.pdf
[2]https://www.mckinsey.com/~/media/McKinsey/Business%20Functions/McKinsey%20Digital/Our%20Insights/Driving%20impact%20at%20scale%20from%20automation%20and%20AI/Driving-impact-at-scale-from-automation-and-AI.ashx
[3] A quantity ‘take off’ (QTO) refers to the process of identifying elements of construction works that can be measured and priced. Drawings and plans are reviewed to forecast upcoming construction costs.