The Government’s recent policy paper ‘Industrial strategy – Building a Britain fit for the future’ has earmarked Artificial Intelligence and data as one of four ‘industries of the future’ – one that can improve lives and boost productivity.[1]
The UK wants to be at the forefront of the AI and data revolution, largely because of their potential to transform business models, sectors and the global economy by identifying better ways of doing complex tasks.
An industry-led AI Council, supported by a new Government ‘Office for AI’, will champion research and innovation, stimulate demand, and accelerate uptake across all sectors of the economy. Construction has been identified as one of six initial priority sectors that will receive support to rapidly adopt next generation AI technologies at scale, helping to enable a productivity revolution.
Where to start?
According to the Government’s Industrial Strategy policy paper, the UK is starting from a relative position of strength. The right building blocks are already in place – strong research institutions, substantial datasets and underpinning technology and robotics companies (eg ARM’s microchips). To capitalise on this position and maximise AI-related opportunities, four focus areas have been established:
- Make the UK a global centre for AI/data-driven innovation – through funding/investment, favourable regulation, and attracting/retaining talent
- Support sectors to boost productivity through AI and data analytic tech – by collaborating with partners to promote adoption and increasing awareness of the advantages of AI
- Leading in safe and ethical use of data/AI – removing barriers to innovation in a fair and safe way. A new advisory body – the Centre for Data Ethics and Innovation – will review existing governance landscape, advise of safe/ethical uses of data and establish data trusts to facilitate easy and secure sharing of data
- Skills development for jobs of the future – Investment in maths, digital and technical skills. Up-skill and re-skill the sectors as the economy changes and employer demand for skills evolves
Despite many excellent and and high-calibre firms operating in the UK construction sector, there is certainly room for significant efficiency and productivity improvements. The AI technologies will help pave the way to improved productivity, closing the gap with other key sectors it has trailed behind.
The productivity problem
According to McKinsey, global labour productivity growth in construction has averaged only 1% a year over the past two decades, compared with growth of 2.8% for the total world economy and 3.6% in the cost of manufacturing.[2] If construction-sector productivity caught up with the total economy, this would boost the sector’s value added by an estimated $1.6 trillion, adding about 2% to the global economy, or the equivalent of meeting about half of the world’s infrastructure need.[3]
There are a number of reasons for lagging construction productivity growth: fragmentation, opacity and misaligned incentives between building owners and contractors are often cited. Small and medium-sized firms – which make up 99% of construction companies in the UK[4] – have limited resources to invest in higher-productivity solutions, which can lead to overruns in cost and time.
Despite the rapidly increasing availability of digital technologies and tools, construction is one of the least digitised industries in the world. The manual nature of the industry increases project supervision complexity, and the absence of digital expertise and technology adoption within the construction industry has also been linked to cost inefficiencies, extended project completion times, poor quality performance, uninformed decision-making and trailing productivity growth in comparison to other sectors.
Embracing AI (and its many sub-fields, outlined below) will enhance automation and provide competitive advantages over conventional approaches.
What is AI/ Machine Learning?
Artificial Intelligence (AI) leverages computers and machines to perform problem-solving and decision-making tasks that typically require human intelligence. At its core, AI is a branch of computer science that aims to answer mathematician Alan Turing’s question – “Can machines think?” – in the affirmative.
Machine learning, which is a subset of AI, uses statistical techniques that allow computers to “learn” from analysed data, despite not being explicitly programmed to do so. Predictions are then created based on an algorithms analysis of this data. For example, question-asking algorithms can track and evaluate progress, identifying schedule risks early on. Any number of inputs can be queried, from weather patterns and machine downtime to previous project data. Risks can then be identified, and stakeholders notified about critical issues that require their attention.
However, there are numerous subfields of AI and construction can benefit from most of them.
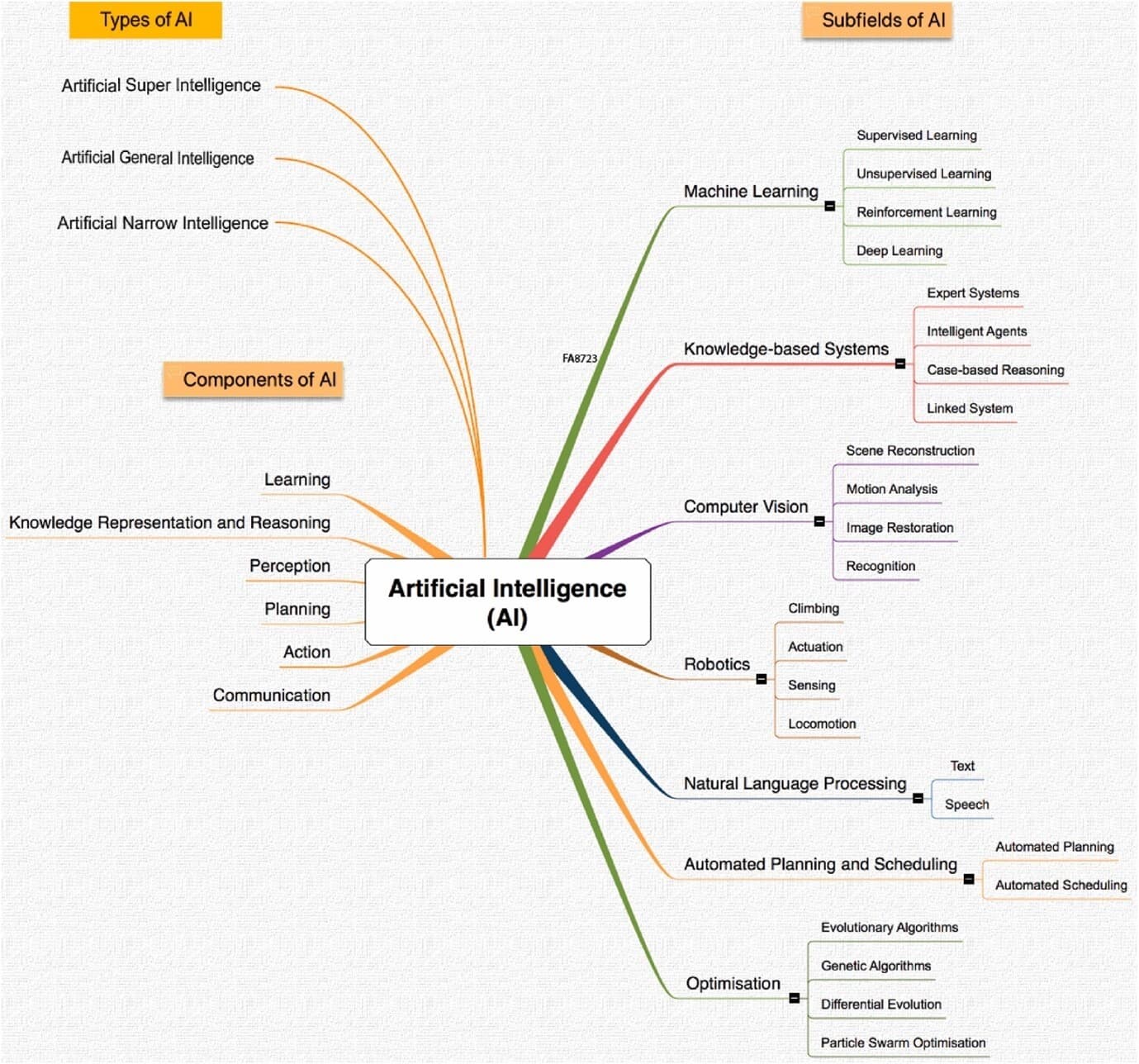
Source: ScienceDirect
There is not a single aspect of the construction process or value chain that cannot be improved by adopting one or more of the AI subfields outlined in the table above. Mountains of data can be scrutinised and used in applications that range from the mundane (eg email filtering) to advanced safety monitoring, quality control and productivity. While it may feel like a far-away concept, several applications of machine learning are already in use.
Subsequent articles in this series will explore the ultimate goals of AI technology in construction and what it can do during different stages of the construction process. We will also explore how the data is collected and how the benefits of AI can be quantified.
[1] The Grand Challenges - GOV.UK (www.gov.uk)
[2] https://www.mckinsey.com/business-functions/operations/our-insights/reinventing-construction-through-a-productivity-revolution#:~:text=Globally%2C%20labor%2Dproductivity%20growth%20in,in%20the%20case%20of%20manufacturing.
[3] Ibid
[4] https://www.fmb.org.uk/news-and-campaigns/key-facts-and-figures.html
[5] Unlabelled data is a designation for pieces of data that have not been tagged with labels identifying characteristics, properties or classifications. Unlabelled data is typically used in various forms of machine learning.