Following OpenAI’s release of its much-hyped chatbot – ChatGPT – in November 2022, many have flocked to experiment with the blockbuster tool, trying to gauge whether a full AI takeover is imminent.
The AI-based conversational interface that can engage in natural language conversations with users and help them with a wide range of tasks (such as answering questions, providing recommendations, assisting with problem-solving) has significant potential, but it can’t do everything.
Some have argued that ChatGPT has the potential to become a biased, disinformation machine, generating sophisticated and plausible-sounding responses or answers that can be incorrect or even non-sensical. Others see it as a new frontier – one that presents opportunities to invent products, services and solutions. While not the intention of this article to assess the possibilities or limitations of ChatGPT, instead we explore some of the challenges that are impeding the wider uptake of AI tools within the construction industry.
The previous article in our AI series explored the goals, capabilities, and applications of AI in construction. The article concluded that, although construction may be slightly behind the curve in implementing AI-based solutions, a shift is coming. More and more resources are being allocated to AI-powered technologies and early adopters have seen significant benefits already, but there are several challenges that are preventing more widespread adoption of artificial intelligence in construction. Some of these challenges include:
- Cultural barriers
- High initial cost of deployment
- Trust
- Security
- Talent shortages
- Computing power
Below, we look at each of these in turn, framing the core issues and considering how some of these might be overcome.
Cultural Barriers
Construction may be one of the least digitised industries, but there are valid reasons why, historically at least, the industry has been slow to adopt new technologies. The risky and costly nature of most construction processes mean that even the smallest of mistakes can have huge implications. As such, there is often little appetite to move away from the traditionally known, tried and tested ways of doing things – even when these innovative technologies have the potential to deliver substantial rewards. However, quantifying the benefits of AI projects with well-defined values can alleviate fear of the unknown.
Unlike other sectors, such as manufacturing, each project or construction site is unique and is constantly changing, meaning AI technologies need to be able to adapt to a fast-changing environment. The work process is complex, generally non-repetitive and depends on factors such as weather conditions, labour and local building regulations. This degree of non-standardisation hinders automation as these factors are difficult to control in an unstructured environment. To win over the industry, AI technologies must be usable on different projects and tested thoroughly enough to convince the supply chain to use them.
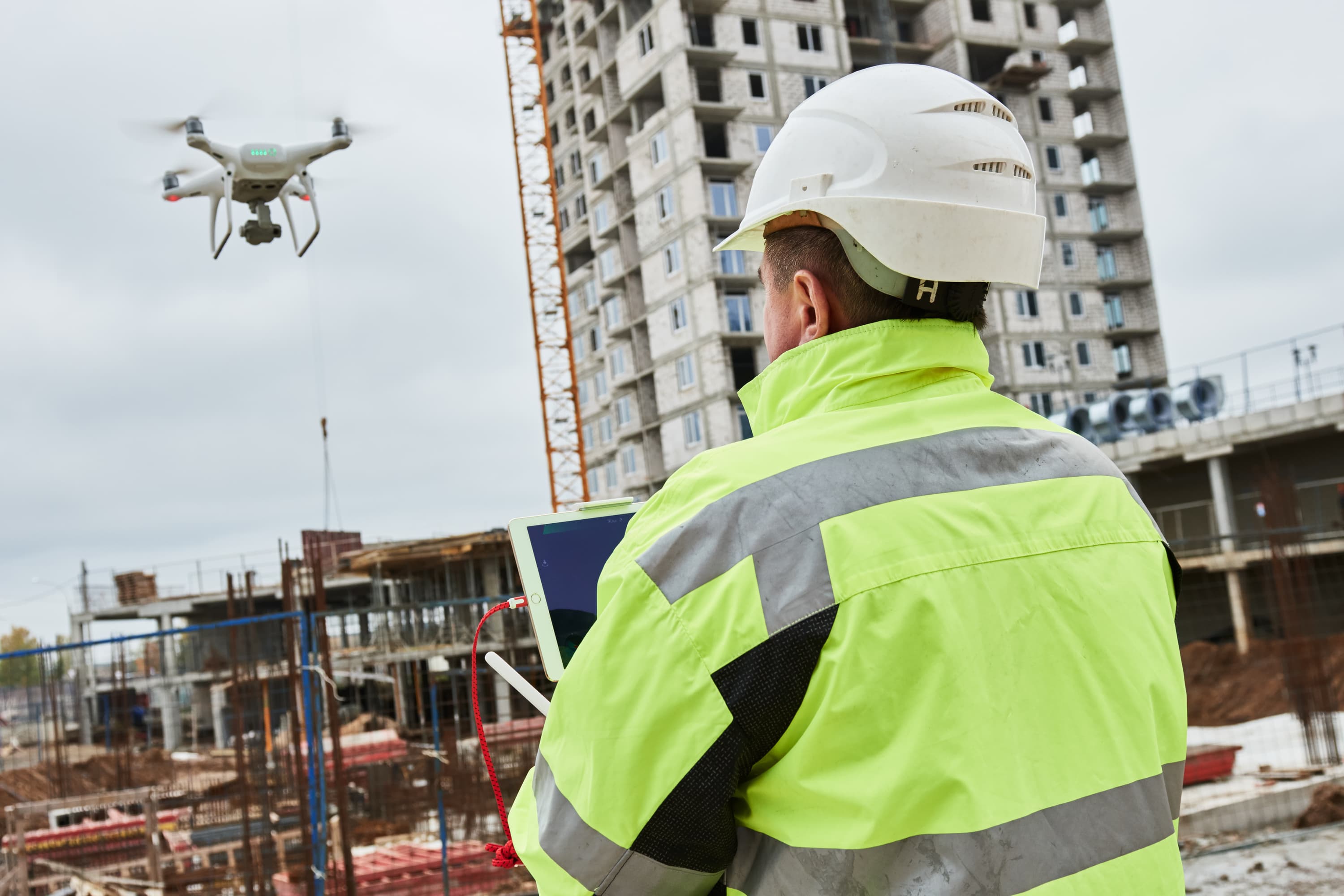
A further barrier is the fragmented nature of the industry. Transitioning to AI models requires compatible design, management, labour practices and site operation practice rather than multi-point responsibility from different project disciplines. Under the traditional model of construction, individual organisations tend to control different phases of the construction process. With little incentive for organisations to share their large data sets, it is a challenge to amass the amount of structured data required to train supervised learning algorithms.
Education becomes the best solution for overcoming cultural problems, generally. By raising the level of awareness, people become receptive and engaged with the potential for positive change AI can bring.
High Initial Cost of Deployment
Initial set-up costs of AI-driven solutions are typically high. There are costs associated with acquiring and managing data, building and maintaining infrastructure and hiring skilled personnel. These costs will be unaffordable for the vast majority of SMEs and sub-contractors that make up the bulk of the industry. Even for those than can afford the implementation costs, it’s important to conduct a cost-benefit analysis that allows firms to identify cost savings and return on investment (ROI). Although the benefits of AI are indisputable, for some firms the business case for investment in AI may not stack up in the short-medium term
Fortunately, as AI technologies evolve and become more accepted and prevalent in the construction sector, set-up and maintenance costs will invariably fall, making solutions affordable for smaller players.
Trust
Trust is also an issue. Most machine learning systems adopt a black-box approach, ie they give you a result or reach a decision without explaining or showing how they did so. The internal processes used and the various weighted factors remain unknown. This lack of transparency makes it difficult to build trust in the AI systems.
One way to address any trust issues is to use ‘explainable AI’ or ‘XAI’ to produce explainable models and enable humans to understand, trust and manage the systems. Some popular approaches of XAI include local interpretable model-agnostic explanations (LIME) and layer-wise relevance propagation (LRP). These make it easier to understand the inner logic or outputs of the model as they map raw information (data) to a meaningful symbolic representation for humans (text). Understanding the rationale will help stakeholders in construction to put their trust in AI-generated predictions or outcomes.
However, XAI does have a computational cost and the more complicated a system gets, the more that system is making thousands or millions of connections between pieces of data. It can therefore still be complicated to explain where a particular decision came from, even when one is able to see the model’s structure and weights.
Security
Security is also a critical issue. Intrusions and exploitations by hackers or cyber criminals can have severe economic and financial implications. Small mistakes in the construction process can often lead to quality, cost and time implications, with a knock-on effect to the project plan. If the data is contaminated[1] (or “poisoned” by injecting malicious samples during operation) by hackers, an entire project can be compromised. From a human safety perspective, compromised security could lead to life-threatening accidents or loss of life. For example, a computer vision system that identifies automated construction equipment may be tricked to mislabel a construction worker working at height.
Consideration needs to be given to how to protect against ‘adversarial machine learning’ – a technique used to manipulate or attack machine learning models by introducing malicious inputs designed to cause the model to make incorrect predictions or produce inaccurate results. Evasion attacks, poisoning and model extraction are common forms of adversarial attack, but there are ways to mitigate the risk of these attacks and improve the robustness and resilience of machine learning models. These include penetration testing (to uncover security gaps), adversarial training (to patch vulnerabilities by training the machine learning model on hostile instances), as well as defensive distillation (which involves training a secondary model to detect and filter out adversarial examples).
Talent Shortages
AI engineers are in short supply globally – particularly engineers with experience in building custom solutions for the construction sector. According to Deloitte, all industries are scrambling to secure AI talent from a pool that is ultimately not growing fast enough[2]. Given the heavy competition (particularly from tech and the banking and finance sectors), hiring is one the most significant issues when it comes to AI integration.
Furthermore, once secured, construction professionals will also be needed to collaborate closely with AI engineers, helping to merge ideas and develop tools capable of tackling the unique challenges faced in the construction industry. This could sap a significant amount of existing resource from construction companies.
One of the best longer-term solutions is to invest in education and training programmes, but shorter-term solutions could involve:
- Encouraging interdisciplinary collaboration (ie between experts in different, but related areas to help to facilitate innovation)
- Leveraging your company’s unique value and purpose to attract AI talent
- Fostering a culture in innovation and experimentation (eg arranging initiatives such as hackathons, innovation labs and idea generation programmes)
- Developing partnerships between companies, universities and governments to share knowledge, expertise and resources. These initiatives can help create a more supportive environment for AI innovation and development and help attract talent
Computing Power
Interruptions to power, telecommunications and internet connectivity on construction sites can pose a significant problem with regards to the use of some AI tools. Sensors and actuators on site communicate critical information that needs to be computed in real-time during the construction phase. Disrupted communications or connectivity can impede the operation of AI tools, but communication technologies such as 5G – which offer greater reliability for construction sites due to its high data rate, reduced latency, energy saving, cost reduction, higher system capacity and massive device connectivity – have been able to solve this problem to a certain extent.
Lack of a Strategic Plan for AI Adoption
Perhaps a combination of several barriers – ranging from a lack of buy-in to a culture not well educated in the practicalities of Ai and digital transformation – AI projects can fail if not planned at a strategic level.
AI must be addressed in strategic business objectives and fit within an organisation’s overall plans for business growth and development. However, many fail to understand the aim and objectives of AI initiatives (whether it be data gathering or uncovering insights) and so AI may not even feature in such growth plans.
Before spending time and resource rolling out costly AI initiatives and pilots, construction companies must have a clear strategy in place. This involves identifying the benefits that AI technologies can bring and linking these to business performance objectives and strategic goals. Any strategic plan will need to identify what success or failure looks like from the outset.
Conclusion
While several barriers remain, progress is being made on construction’s AI journey. Construction has become increasingly digitised in recent years – a key prerequisite or critical enabler in deriving value from AI. A strong digital backbone will be necessary to train AI systems and build effective models, but additional foundational enablers are required to derive value from AI at scale.
AI can be difficult for businesses to trust, control or monitor. As such, many of these barriers derive from fear of the unknown or the absence of a sophisticated strategic plan for AI. However, talent gaps, security concerns and high initial deployment costs are also very real issues that need consideration before planning an AI project.
In our next article, we explore how business and technology leaders in construction can plan and prepare for AI projects, with a particular focus on what steps need to be taken prior to the deployment of AI solutions (addressing issues such as data discontinuity, inconsistency, and inaccuracy). Based on G&T’s recent conversations with one UK-based construction management software provider, we summarise what it is doing to forecast outcomes of construction projects, helping them to be built on time and on budget.
[1] There are a large variety of different adversarial attack categories that can be used against machine learning systems (poisoning, evasion, and model extraction attacks) but most usually aim to deteriorate the performance of classifiers on specific tasks, essentially to “fool” the machine learning algorithm
[2] https://www2.deloitte.com/us/en/insights/industry/technology/ai-talent-challenges-shortage.html